2022 AWS re:Invent Recap and Enterprise Themes for 2023
December 23, 2022
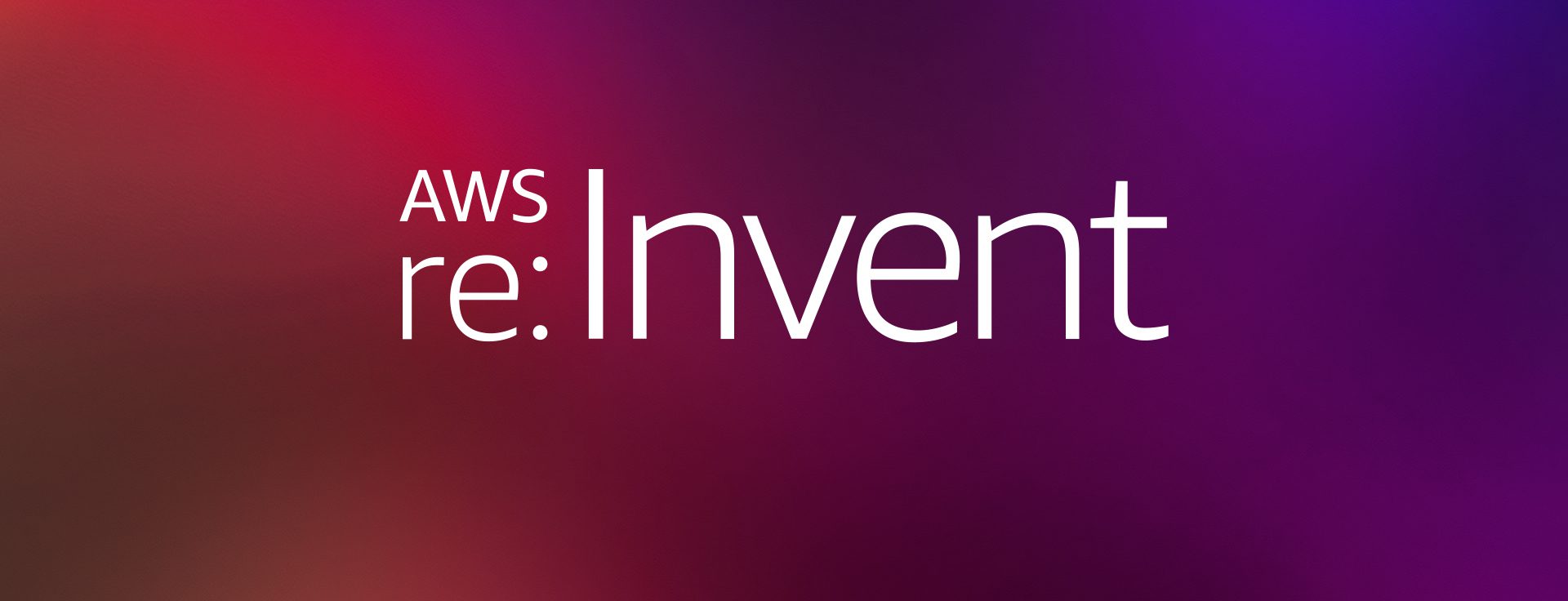
After staging a virtual-only event in 2020 and a scaled-down version in 2021, AWS re:Invent returned in full force last week in Las Vegas with over 50,000 attendees for the 11th edition. Here are some of the major announcements and our views on some of the relevant themes we’re tracking for 2023.
By Maura Kopko, Dalton Ang, Raj Sharma, and Suman Natarajan
After staging a virtual-only event in 2020 and a scaled-down version in 2021, AWS re:Invent returned in full force last week in Las Vegas with over 50,000 attendees for the 11th edition. This year’s conference featured an excellent list of industry-specific experts and breakout sessions. However, relative to past years, this year’s AWS had fewer blockbuster product announcements; instead, there were more capabilities and product initiatives that were focused on Day 2 cloud activities, i.e. efficiently managing cloud workloads and data at scale. Below are some of the major announcements and our views on some of the relevant themes we’re tracking for 2023.
Infrastructure Efficiency and Cost Optimization
Optimizing cloud computing and infrastructure costs, also known as “FinOps” or financial operations, has become imperative to most organizations. In this current macroeconomic environment, companies are looking to cut down on costs where possible and overprovisioned cloud resources are a great place to start. On Monday night’s keynote Peter Desantis announced three new Amazon EC2 instances powered by three new AWS-designed chips that offer customers even greater compute performance at a lower cost. With each successive chip, AWS delivers a significant improvement in performance, cost, and efficiency, giving customers even more choice of chip and instance combinations optimized for their unique workload requirements.
Our portfolio company, Zesty, is particularly excited for this announcement because it supports their obsession with optimization. Zesty is a cloud cost optimization platform that helps enterprises optimize their infrastructure spend and only consume the resources that are needed to run their applications, reducing time and waste. Zesty’s machine learning algorithms observe application behavior over time to understand and predict their usage and in real-time buy and sell cloud instances to fit the needs of the application. This replaces the need for DevOps teams to continuously monitor their cloud environment and adjust their instances and resources to manage their costs.
Modern Data Stack and Bridging Across Data Silos
One of the biggest product announcements at this year’s AWS conference was its move towards a Zero-ETL future on their cloud platform, where they aim to eliminate the need for customers to build and manage pipelines to “extract, load, and transform” data across data services in order to perform holistic analytics. Currently, if customers want to perform analytics across data that is stored across different silos, they need to build ETL data pipelines to physically move data to a single location. Without the need for ETL customers no longer need to bear the overhead of managing a pipeline, but also they needn’t pay twice for storing the same data and reduce their time to insights. Their preview to this ambitious undertaking was a deeper integration between their transactional datastore, Amazon Aurora, and their analytics data warehouse, Amazon Redshift.
The challenge of data sprawl is exactly what our portfolio company has been addressing, with their federated SQL query engine. They are providing a single point of access to allow enterprises query their data regardless of where it resides, both on-prem and now in the cloud, with their Galaxy offering. The company recently launched Data Products capabilities, which allow enterprises to gain better control over their distributed datasets, with data and schema discoverability features and granular access controls for intelligent collaboration.
Data governance, collaboration, and operations will continue to be a core area of focus for our team as enterprises are continuing to evolve to a modern data stack. We also expect to see new opportunities for companies to further refine the semantic data layer.
Democratization of Data Science
Another theme was focused on reducing the friction to stand up and scale data science initiatives in larger enterprises. Several product announcements include AWS integration with Amazon Redshift, AWS DataZone and Amazon QuickSight, which are all aimed at eventually collapsing the OLTP/OLAP divide and making machine learning tools more accessible to everyday users.
From demand forecasting to document processing and fraud detection, machine learning shows a tremendous potential for driving automation and improving process efficiency. However, companies still face challenges when attempting to adopt machine learning initiatives. One challenge is the difficulty of integrating data across the entire data lifecycle, which makes it challenging for companies to build and operationalize machine learning models.
Broadly speaking, the data science workflow can be thought of as containing about half a dozen steps, including data ingestion, data integration, feature engineering, model testing, deployment, and model monitoring. Only a handful of tools attempt to play across this entire lifecycle. One such company is our portfolio company, DataRobot, which offers an end-to-end platform for machine learning. DataRobot aims to democratize access to machine learning, and we’re excited to see new startups providing collaboration and MLOps continuing to remove barriers to adoption to make data science adoption more widespread.
Return of Severless
Serverless is a cloud-native development model that allows developers to build and run applications without having to manage servers. AWS introduced Lamda functions back in 2014 with significant fanfare, but adoption to date has failed to live up to the hype as mainstream cloud adoption was still relatively early. In this year’s re:Invent conference, AWS launched several features that built on top of the core Lambda functions to simplify developing, orchestrating, and monitoring event driven applications. New features include telemetry for observability, EventBridge and its Scheduler, to simplify creating and managing tasks at scale, and Step Functions which leverage serverless functions to perform data basic data transformations.
While the first generation of Lambda functions were designed for developers to use them directly, we’re excited to see new startups leveraging the versatility of serverless to power new use cases, such as Vendia providing trusted data exchange, and companies that are helping building blocks to support more efficient application development.
Realization of Multi-cloud
As enterprises continue to manage against the risks of cloud lock-in from a security and performance perspective, we believe platforms and solutions that help manage and improve multi-cloud businesses will be a key area of focus for the years to come. Key to realizing a multi-cloud future is an intelligent and high performant network to traverse across services and infrastructures. Moreover, as enterprises accept the new normal that distributed work forces across different geographies are here to stay, the need for distributed application architecture and infrastructure is no longer optional and is needed for traditional enterprises. With the arrival of real-time analytics, AI/ML, and 5G, we expect to see adoption of edge computing to continue to rise as the ability to locally process data and securely deliver insights across cloud environments is more readily feasible.
Perimeter81, a B Capital investment, builds secure remote networks based on a zero-trust architecture, eliminating the need for legacy solutions like VPNs and firewalls. We’re excited about other startups building on this theme, including Prosimo that build and automate multi-cloud networking infrastructures completely, improving user to application and application to application performance and security.