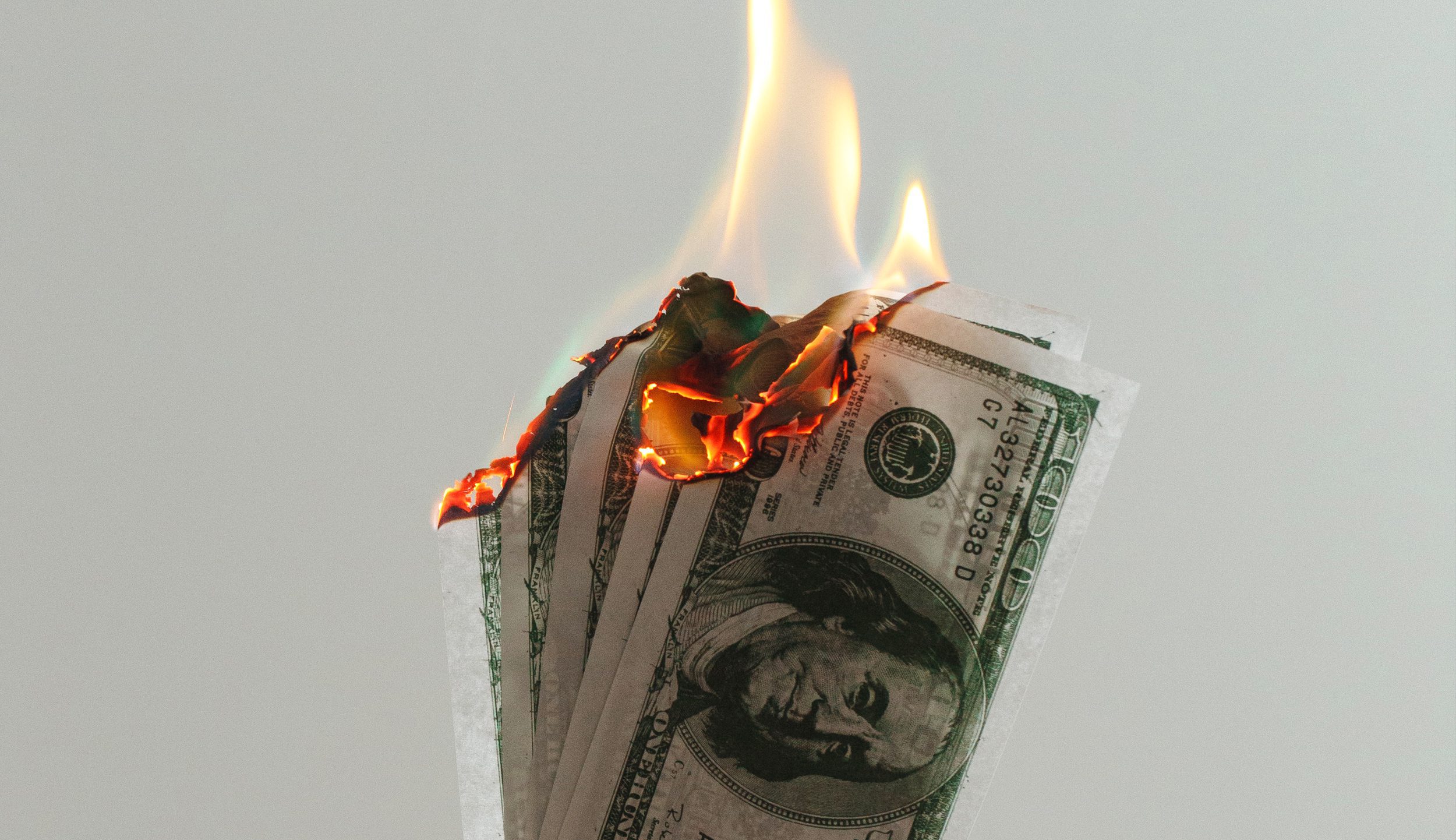
By Mike Fernandez , B Capital Group
Banks have faced a difficult regulatory environment in the aftermath of the Global Financial Crisis. Since 2008, regulators in developed economies have levied over $321B in fines against banks, and banks are struggling to keep up with the over 50,000 regulatory changes that occur each year. In response, banks have grown compliance headcount significantly, with compliance employees now representing 2–3% of all FTEs at large universal banks. The majority of this headcount growth is designed to target financial crime compliance, which we now estimate to be a $4B cost center for global and large regional banks in the United States and Europe, not including fines.
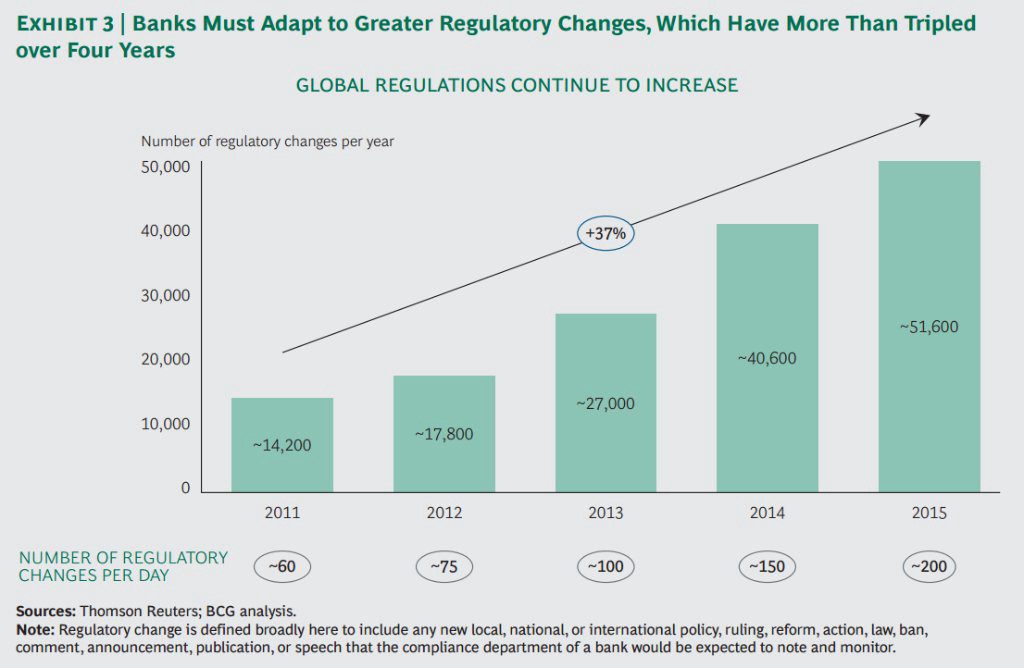
Many of the day-to-day tasks involved in maintaining regulatory compliance in fighting financial crimes are highly routine and repeatable. Legacy systems such as NICE Actimize and Oracle/Mantas flag suspicious transactions, which are then manually reviewed by compliance personally before making necessary regulatory filings. Unfortunately, these systems are based on rigid rules that do not adapt to the changing behaviors of criminals or the broader context of activity. As a result, an estimated 90% of flagged transactions are false positives, driving unreasonable amounts of human review.
Fortunately for banks, a technology solution to these spiraling costs is appearing on the horizon. Compliance is an area which is particularly well suited for automation through machine learning. Banks can use machine learning to develop predictive models which are more accurate at detecting problematic transactions than the current heuristics-based methods, dramatically reducing the false-positive rate. Because these models are self-updating, they can also keep up with the changing methods of bad actors faster than regulators can publish new guidelines and banks can update their rules-based systems. Additionally, portions of the transaction review process can be automated, requiring only cursory human review of transactions.
There are still some technology and regulatory hurdles to be overcome before new machine learning software can fully replace incumbent systems. One of the challenges inherent in the financial services market is the sensitivity of data, both for regulatory and competitive reasons. Most machine learning approaches today require centralizing data in the cloud for analysis. Multinational banks that are subject to an international patchwork of data policies find it difficult to even centralize data within their own company. Transferring all transaction data to a third party in the cloud to be intermingled with competing banks’ data is a non-starter.
In the age of machine learning, having access to a large, unique dataset creates competitive advantage. Having machine learning models that remain siloed within individual banks could lead to a world in which we see increasing returns to scale from compliance activities. Large international banks with complex transactions, which may be more susceptible to money laundering, would develop smarter models over time as their systems see more and more bad transactions. Smaller regional banks would end up with less effective models, and may inadvertently have more risk of compliance failure despite a lower overall number of bad transactions. While individual banks may like this outcome, it is less than ideal for solving the social issue of money laundering.
An emerging concept in machine learning called federated learning presents an intriguing solution to this problem of returns to scale in regulation. In federating learning systems, training data is kept in local environments (whether that’s an individual’s mobile phone or a bank’s internal systems), removing the need to duplicate data into the cloud. The local environment downloads the latest model from the cloud, runs it on local data, and then requires that only new model updates be recentralized in the cloud. No underlying data is at risk of being shared globally. Therefore we are seeing emerging use cases for federated learning in diverse areas ranging from smart mobile phone keyboards to Industrial IoT analytics, where the amount data generated by industrial sensors can overwhelm meager connectivity.
In a federated learning system designed to identify money laundering transactions, all banks using the system would benefit from each other’s transaction data in building more capable models, without exposing their own raw data to competitors. This type of system might be more appealing to regulators, as it would give all banks equal footing to fight financial crime. At the same time, it would unlock network effects for the startups that adopt this approach, creating a potential winner-take-all situation in the market and improving returns for the leading player.
The market for machine learning-based compliance software is still in its earliest stages. Most startups in the space are still exploring pilot projects with banks, and there are few commercial deployments to date. Many of the underlying technology problems have yet to be fully solved. Both financial institutions and startups need to actively engage with regulators to ensure a smooth path for acceptance and adoption. However, given the immense costs to banks of compliance failures, we believe that this remains one of the most promising use cases for machine learning in a financial services back-office environment.