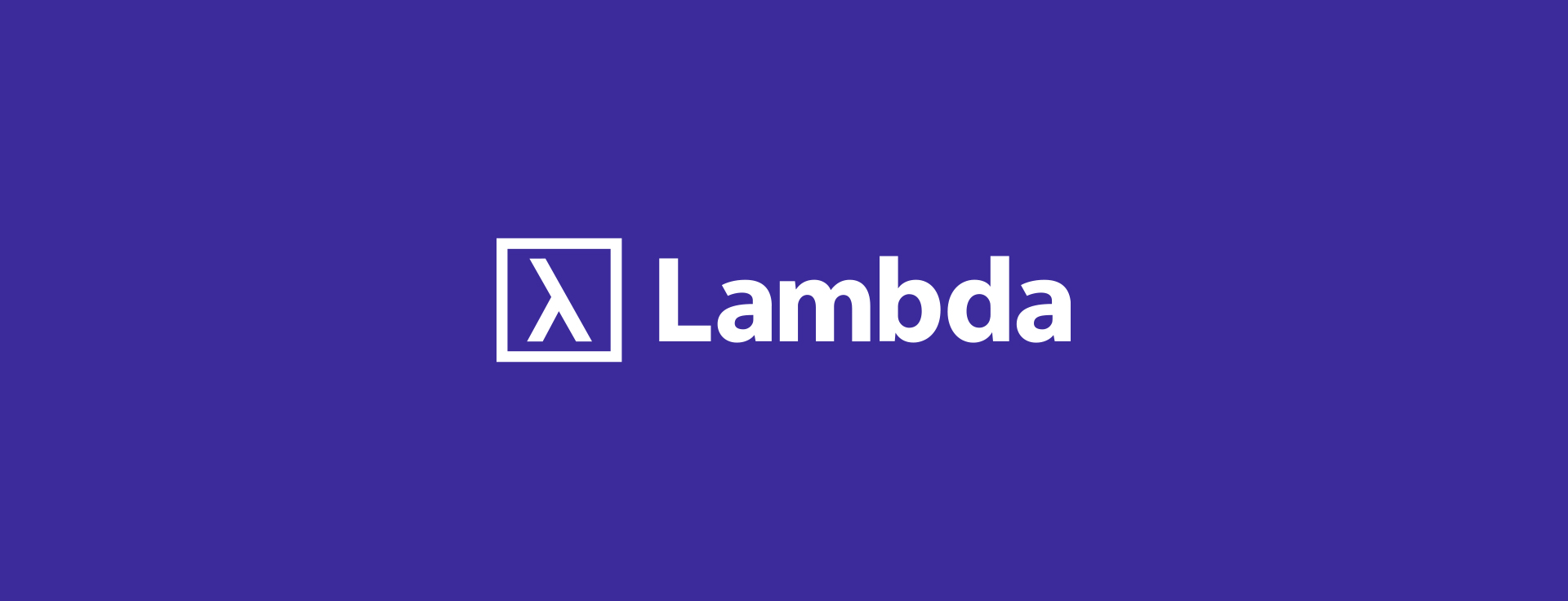
By Rashmi Gopinath, Suman Natarajan, Brian Alter, Jeff Goldsmith
Background / The Problem
The advances in deep learning mark one of the most transformative technology trends of our time with the potential to reshape entire industries and alter how humans engage with technology. Since its public release in November 2022, Open AI’s Chat GPT captured the world’s attention as to the power and potential of AI and Generative AI applications. In 2023 alone, we witnessed the emergence of several hundreds of AI startups, with investments in this space exceeding $50B[1]. The global race to develop, train, and harness AI insights has drawn participants from a wide spectrum, including individual experimenters, creatives, startups, research institutes, traditional enterprises, and tech giants. Notably, according to Gartner, market adoption is still grossly under penetrated but set to expand at a staggering rate. They forecast that by 2026, over 80% of enterprises will have integrated Gen AI APIs and models into their production environments, a remarkable leap from the less than 5% adoption rate seen in 2023.
The not-so-old maxim that “every business is a software business” has now evolved to “every business is an AI business”. To make democratized AI a reality, widespread access to high-performance and cost-effective compute, particularly GPUs, is crucial. As LLMs and Gen AI models require orders of magnitude more processing power than traditional models and as businesses hasten to gain a competitive edge with Gen AI, the demand for GPUs has skyrocketed. Supply chain disruptions and chip shortages have heightened competition among enterprises and hyperscalers, all vying for allocations from semiconductor leaders Nvidia and AMD. TSMC, the chief chip manufacturer, predicts that these shortages will persist into 2025[2]. Even companies offering GPU alternatives or specialized AI chips are encountering delays, as they are also affected by shortages in crucial components and are dependent on TSMC’s limited resource capacity.
Ultimately, access to GPUs depends on an enterprise’s chosen infrastructure provider – who dictates allocation wait times, compute costs, SKU availability, ease of implementation, and performance quality. The major cloud providers have quickly scaled up their GPU offerings to meet the growing demand. However, customers complain about the high cost and low availability of the best SKUs – with some customers waiting months for GPUs, only to find sub-par performance due to inefficient configurations and software bloat. Several dedicated GPU clouds have emerged to improve on the cloud players’ shortcomings, although not all were purpose-built for AI use cases, impacting performance quality. Also, some of the specialized GPU vendors solely cater to the three hyperscalers who place enormous orders, taking up most of GPU capacity and squeezing out other customers. Finally, hyperscalers fail to offer a cost-effective, on-prem solution. Organizations in regulated industries, ones with strict data residency requirements, and increasingly nations seeking AI sovereignty[3], must leverage their existing data centers or build new GPU datacenters. In doing so, they take on the enormous burden to directly purchase, set up, and maintain the GPU hardware and software all of which are not part of their existing team’s skillset.
Overview of Lamba
Founded in 2012, Lambda Inc. has over a decade of experience building AI infrastructure at scale and has amassed over 100,000+ customer sign-ups on Lambda GPU Cloud. As a launch partner for NVIDIA H100 and GH200, Lambda GPU Cloud is chosen by AI developers for the fastest access to the latest architectures suited for training, fine-tuning and inferencing of Generative AI, Large Language Models, and Foundation Models.
Lambda is led by AI engineers and only delivers solutions for AI workloads. Their diverse range of offerings sets them apart from all other infrastructure companies, allowing them to serve virtually any AI developer, thus truly enabling democratized access to AI. Lambda sells GPU solutions across every compute modality, from laptops and workstations to large on-prem clusters and Cloud contracts. For on-premise customers, they sell pre-built servers and racks for datacenters, including Nvidia’s latest DGX SuperPod, and offer managed co-location in their own datacenters. Their cloud platform allows on-demand GPU rentals, reserved instances and features to come that will continue to set the bar for high-performance and easy-to-use AI infrastructure.
All their form factors come pre-configured with their proprietary software stack, Lambda Stack, that includes popular deep learning integrations and frameworks, ensuring a consistent experience to build, train, and deploy models. As a dedicated AI infrastructure provider, Lambda can better forecast their GPU needs, thus offering better access than cloud providers. Also, Lambda’s highest tier of partnership with Nvidia allows them to deliver the latest-and-greatest, premium GPUs to customers in weeks, in contrast to the months it takes for cloud providers. Finally, their hardware expertise enables them to optimize their SKUs for operational efficiencies at large scale, and hence the ability to offer the most competitive prices on the market.
The current iteration of Lambda was the result of the founders seeking to solve their own infrastructure challenges. Founders and brothers, Stephen and Michael Balaban, CEO and CTO respectively, co-founded Lambda to address their frustration with rapidly rising cloud spend when they were building an AI software company offering facial recognition software and were determined to find a more cost-effective solution. They built their own GPU infrastructure replacing their $40k/month AWS bill with just $60K of capex spend. In 2016, as the field of data science started to expand, the team recognized the growing demand for dedicated and affordable AI infrastructure and pivoted the business to sell GPU workstations and servers, which they successfully built into a profitable business. In 2022, they reinvested profits from their data center business to fund their expansion into GPU cloud offerings that significantly accelerated their growth.
Why We Invested
As part of B Capital’s AI thesis, we have been tracking Lambda for the past couple of years and have been impressed with their thoughtful execution as they built and scaled a profitable data center business serving over 2,000 customers, to then launch a GPU cloud business, which now serves several Fortune 100 enterprises, research institutes, and AI startups. Stephen and Michael have clear founder-market-fit to build an AI infrastructure business. Stephen started his career as a data scientist, building a neural networking company that was sold to Apple in 2013. Michael has deep expertise in building out highly scaled infrastructure, leading the effort to scale Nextdoor’s backend to support over 100M users.
Their Day 1 focus on AI and ML has earned the trust of thousands of AI Developers, ML Engineers, and Researchers from leading institutions like Microsoft, Amazon Research, Kaiser Permanente, Stanford, Harvard, Caltech, Raytheon, Airbus, and the Department of Defense. Lambda deeply understands the needs of this customer base and has optimized their networking, storage, memory, security, and software architectures to best serve AI deployments at tremendous scale.
Generative AI represents one of the most momentous tech advancement opportunities of our time, and we see a long term need for dedicated AI infrastructure to power this new normal. Over time, we expect GPU capacity to open and for models to become more efficient. However, we believe that Jevon’s Paradox applies to this market – i.e. lower cost of GPUs will catalyze even greater consumption, accelerating mainstream adoption and unlocking ever more use cases.
Lambda is uniquely positioned to address the AI momentum and spearhead efforts to democratize access to AI. We are thrilled to partner with Stephen, Michael, and the Lambda team as part of their $320M Series C fundraise and look forward to supporting Lambda as they continue to build the foundational infrastructure that will shape the future of AI.
[1] Artificial Buildup: AI Startups Were Hot In 2023, But This Year May Be Slightly Different (crunchbase.com)
[2] TSMC sees AI chip output constraints lasting 1.5 years – Nikkei Asia
[3] Jensen Huang Says Demand for Nvidia Will Grow as Countries Build AI Systems – Bloomberg